When I founded Chef Robotics in 2019, the question I had was what technology would have the biggest impact on the world in our lifetimes. It felt clear that it would be AI. But it also seemed clear that AI’s biggest impact would not be in the purely digital world but rather in the physical world–which represents 90% of GDP–in the form of Embodied AI through robotics.
But despite all the buzz around the advancement of AI in robotics in recent years, it felt like nobody had really cracked the code and scaled deployments of production-grade intelligent robots at external customer sites at a massive scale. It felt like the potential was vast, especially when you consider that the human labor industry is the largest industry on planet earth (at around half of all GDP). And yet there hadn’t been anyone that had ushered in the intelligent robotics revolution like Tesla had for EVs, Intel had for the integrated circuit, Facebook had for social networks, Uber had for physical-world marketplaces, or OpenAI had for LLMs. So I talked to dozens of robotics founders, customers in various industries, and investors to learn what had happened to those companies and what we could do differently. I wrote some of my learnings here.
Over the past few years at Chef, we’ve worked in stealth mode. We’ve kept our heads down as we developed our first-of-its-kind food manipulation AI and robot system, hired a world-class team of engineers and operators who have previously built complex AI-enabled systems at top Silicon Valley companies, built strong relationships with industry-leading customers, and brought on some top tier investors. We’ve now deployed our robots in production environments across six cities in North America. We’ve reached the milestone of completing 20 million servings in production, and have achieved more volume of food made in production than any other intelligent food robotics company. Now, with a renaissance in AI, we find ourselves uniquely positioned as the market leaders in the food robotics space and are ready to share our story and the details of our operations for the first time.
Back in 2019, after we had decided we wanted to focus on Embodied AI, the question we asked next was what industry do we start in? We decided to start in the American industry most in need of AI-enabled robotics: the food industry. Why? Our first criterion was what is the largest market we could go after? We learned that the two largest markets in the U.S. by labor force – according to the Bureau of Labor Statistics (BLS) – were Nursing Aids/Personal Aids and Retail Salespeople, both jobs that we felt were not tractable by AI anytime soon. The food industry was the third largest, and from a labor force size perspective (a good proxy for the market size for AI), we became convinced that the food industry was the largest market tractable for AI.
The next question was does the food industry have a hair-on-fire problem to solve? We learned that it does both from data and anecdotally. With 1,137,000 unfilled jobs – the largest labor shortage of any US industry according to the BLS – and an annual staff turnover rate of 150%+, it became clear that people in the US no longer want to do dull, dangerous, and dirty jobs in food preparation and production. This especially posed a challenge for food manufacturers who wished to keep their supply chains onshore, as they struggled to meet the challenge of high production demand coinciding with their industry experiencing the #1 labor shortage in the US. Many were and are reluctantly considering moving parts of their supply chain off-shore, which poses an existential threat to the US food supply chain.
At this point, it became clear that there was 1) a big market and 2) a big problem to solve. So the next question was what’s the right task within a commercial kitchen to start with? At this point, we had talked to everyone in the food industry we could talk to: food trucks, fast casual restaurants, ghost kitchens, hotel operators, stadiums, hospitals, K-12 schools, universities, prisons, airports, airline caterers, meat packers, and food manufacturing companies. What we learned was that each of them broadly divided their operations into three steps: prep, cook, and plating. Interestingly, we learned that assembly or plating the food (what you visually see at Chipotle) is 60-70% of the labor in a non-fine-dining commercial kitchen. The reason is that the labor to do food prep scales sub-linearly with volume since there’s a lot of traditional food processing equipment that works well (like Robo Coupe and similar industrial equipment). And cooking you can do in batches so a few people can cook for hundreds. These early prospects told us and we agreed that the best place to start would be to help food companies overcome the labor shortage and increase production volume by focusing on food assembly.
Now the question was: why doesn’t traditional automation work? We learned that automation has played a role in the food industry for quite some time (e.g., the dispensers you may have seen growing up on How It Works). The issue with traditional automation is that it’s not flexible. Until now, robots have only been built to automate one specific task each; this works well for “low mix” production lines where each line has one specific job to complete but falls short in “high mix” environments where food companies must accommodate hundreds of SKUs and create custom meals. In these environments, traditional automation simply doesn’t work. As a result, these food companies have had to rely on hiring millions of people to assemble meals manually. In the commercial kitchens we’re all familiar with – think fast casuals, hospitals, stadiums, food trucks, prisons, and event centers – you see dozens of people scooping food with ice cream scoops on a makeline. In industrial kitchens, the process is quite similar except that the plating is done on long conveyor lines in rooms kept at temperatures of 34° Fahrenheit. In either case, Americans are reluctant to do this tedious and labor-intensive work.
So we defined our vision. What if we could help food companies overcome this labor shortage and increase production volume by deploying AI-enabled robots in every commercial kitchen in the US, and then the world? This was compelling. Hard. But exciting. Something that could put a dent in the food industry.
From there, we decided to raise our first venture capital, hire an early team, and get to work. The good news from a technical execution perspective was that with the power of AI, we could take off-the-shelf hardware – collaborative robot arms, depth cameras, CPUs, GPUs, and other sensors – and make it flexible enough to provide labor supply in the form of robotic automation, helping food companies meet demand.
Of course, the issue with building a real-world food manipulation AI is there’s a cold start problem: to have a highly capable AI, you need training data; in purely cloud AIs (like LLMs), you can simply download the open internet. In self-driving cars and in rigid body manipulation (e.g., mixed SKU palletizing or bin picking), you can often use simulation to generate the data. But in the food industry, there’s no off-the-shelf training data and there are no physics-based simulation engines that work well since food is deformable, sticky, and wet. In other words, to generate valuable training data, we learned that you need to deploy robots in production environments. And given everyone preps and cooks food differently (something that was made today will likely never be made the exact same way again), we realized Chef needed to find the fastest path to deploy robots at a wide variety of facilities to ensure the robots interact with a large breadth of foodstuffs and to simply just deploy a large number of robots in the world.
To generate this training data, we decided to initially deploy robots in food production and manufacturing environments (not restaurants) where Chef could partially automate a food operation and thus add value in production to customers without requiring 100% full autonomy from the get-go (this is also our Thielian “secret”). We built Chef’s systems on modern advancements in deep learning to make them highly flexible and adaptable enough to “pick” and plate almost any ingredient; this makes them an ideal solution for assembling or plating food.
Today, we have produced over 20 million servings and have a fleet of AI-enabled robots deployed all over North America. These robots are actively generating ROI for our customers. For example, we’ve helped our customer Chef Bombay reduce standard deviation by 30%, reduce food giveaway/wastage by 88%, increase labor productivity by 33%, and increase throughput by 9%. At Chef, we believe one of the best metrics of success for AI-enabled robotics is a customer buying more robots – for a customer to do this, the robots have to work and generate ROI.
The great news is that scaling within our existing customers allows for even more food manipulation data collection. And, success with our existing customer base means that when we chat with new customers, we can promise high utilization from the get-go (we can already do a high proportion of their foodstuffs), further accelerating our flywheel.
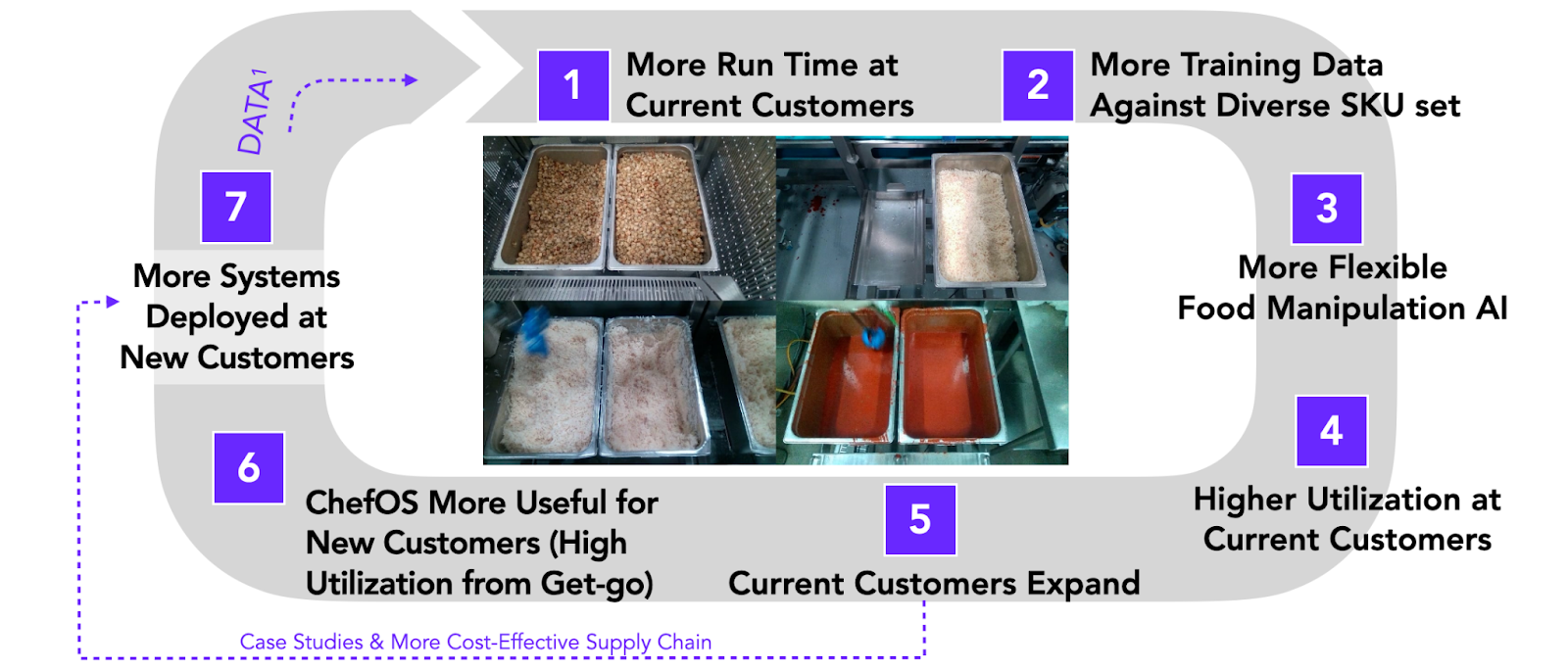
The real-world interaction gained from having robots deployed in production environments is what will allow us to perfect our core AI models for food manipulation. These models continue to evolve as we deploy more robots that see more depth and breadth of ingredients; these robots essentially act as data ingestion engines to feed our AI models. As the AI becomes more and more autonomous – handling more ingredients and ever more of the long tail edge cases – we can then leverage these systems to go from applications like industrial food production (high volume, high mix) to ghost kitchens (mid volume, high mix) and ultimately, to deploying AI-enabled robots in every commercial kitchen in the country (low volume, high mix). In other words, once the AI learns how to manipulate shredded chicken in a large industrial kitchen, it can leverage the same model to manipulate a similar shredded chicken in a commercial kitchen. At every step of the way, Chef is becoming more flexible and able to handle a higher number of edge cases, ultimately adding more and more value to a wider range of customers and applications.
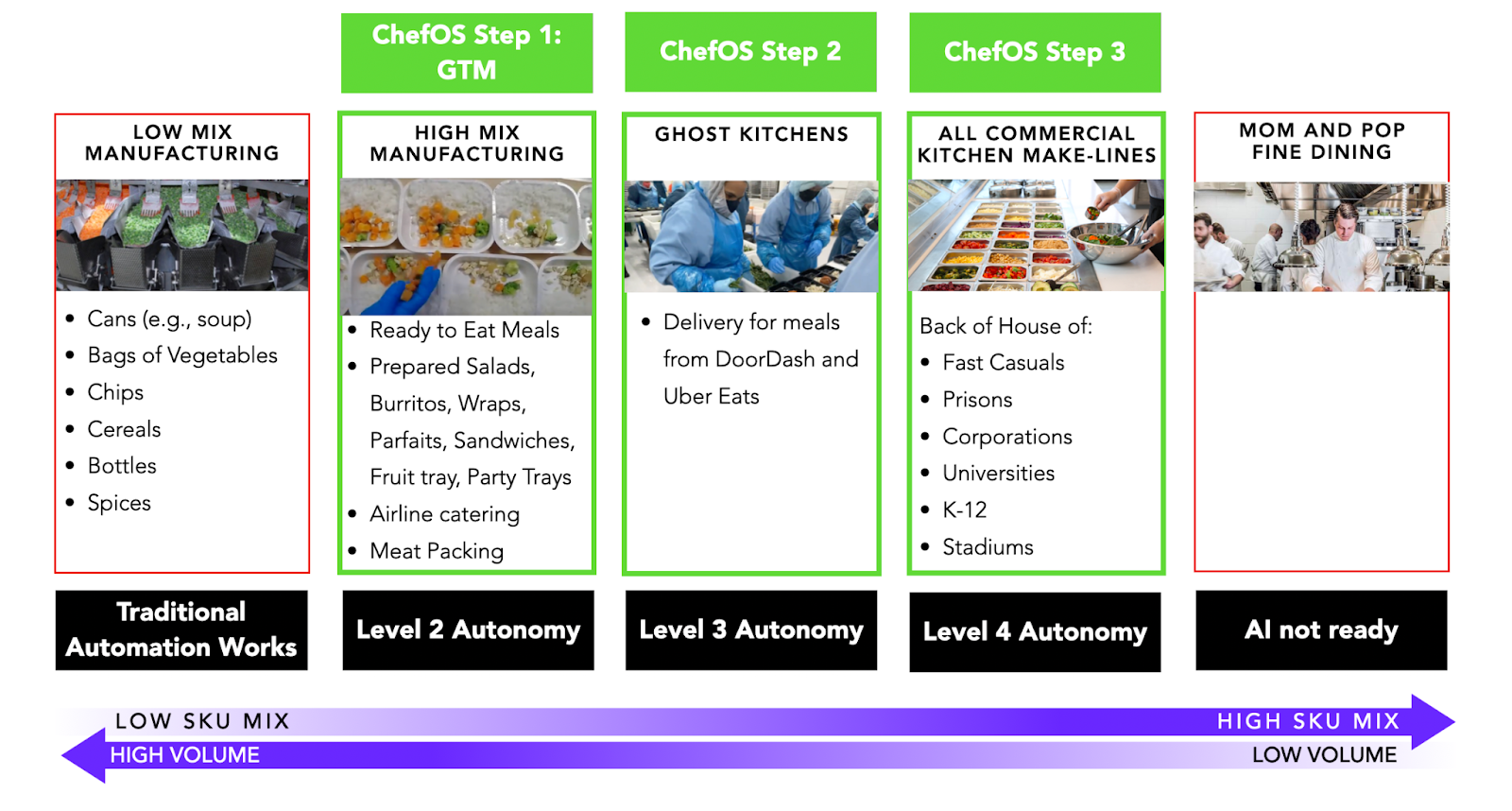
What’s exciting is that modern advancements in AI are further accelerating ChefOS’s flywheel. Today, Chef is leveraging transformer-based diffusion policies and has created a new method to teach the system new tasks (e.g., new classes of ingredients). Before, this process required writing code (and potentially even designing a new utensil). Now, anyone can do “demonstrations” and Chef leverages imitation learning to teach the robot the new task. This is similar to the technology that powers Sora or DALL-E except that rather than predicting the next image/pixels, we're predicting the next robot action. This technology allows us to accelerate the flywheel further since it reduces the time it takes to onboard new classes (and more ingredient coverage drives robot utilization). We’re now working towards moving from a rule-based ML system towards an end-to-end system that transforms pixels to robot actions. We’re on our path towards creating a Generalized Food Manipulation Model; this is only possible because of the 20M servings of in-the-field production data (which is necessary to train a embodied AI foundational model).
Over time, not only will we scale from high volume operations to lower volume operations (like fast casuals), but we’re also going to work to enable ChefOS to help with many other applications in commercial kitchens. Given all the production data we’ll have, we’ll work to have ChefOS help with other facets of food operations: for example, placement weight analytics, QA/QC for placement accuracy, line monitoring and metrics, line scheduling and production planning, piece picking of countable items, and systems that help with prep and cook too. We hope to become a more fundamental part of the day-to-day operating systems that food companies use to run.
So although we’ve chosen food production as a starting point, we see it as just that… a start. Sometimes it makes sense to sell books before selling everything even though the mission is to be Earth's most customer-centric brand (a la Amazon). Or to build an on-demand taxi service for the wealthy in San Francisco before making it cheaper and expanding it to everyone even though the mission is to move anything, anywhere (Uber). Or to build an expensive electric sports car before building a car for the masses even though the mission is to accelerate the world's transition to sustainable energy (Tesla). So while our ultimate goal is to free humans from mundane work so they can focus on more fulfilling endeavors, that starts with placing robots on food production lines first.
Further, once we define the principles that comprise the playbook on how to scale intelligent systems, the learnings aren’t limited to food; learning how to sell before building, hire the best, build a scalable product, build the software infrastructure needed to support that product, price and market the system, have extremely great customer success, have repeatable sales motions, and land and expand within a customer are all lessons that can be applied across industries. The progress of most robotics companies is halted by the same things over and over again. So if we can learn how to overcome these recurring challenges once (and have access to proprietary data of how to do deformable material manipulation – a problem that’s a lot more complex than rigid body manipulation), we have a strong chance of being able to repeat our success. With the right formula and training data sets in hand, the opportunities to expand into more non-food, generalized dexterous manipulation of day-to-day items in environments like hospitals, warehouses, factories, construction are endless. This last part is not a 1 year or 5 year or 10 year goal. This is a 20+ year goal. The really great things in history — one day delivery, the making of the steam engine, scaling the Internet to the masses, scaling electricity, the airplane, the computer, the automobile — never happen fast. But over the long term, we’re building Chef so that this company, its people, processes, and its culture can help bring forth a future where humans are free to focus on the jobs that they enjoy the most and do the best, and machines can handle the dirty work.
Of course, today we’re focused 100% on food manufacturing. If you’re a food manufacturer and think Chef could help, please get in touch!
And if you’re looking to help accelerate the advent of AI in the world and join our mission, please also reach out!