Quality assurance plays a crucial role in the food preparation industry. Facilities that prepare packaged meals must meet rigid quality and safety standards, or risk posing a threat to public health and being shut down. Quality assurance practices are often very time consuming, and lack a targeted approach. Often an entire production run’s worth of meals will have to be recalled or thrown out when an incident occurs on a production line.
With these challenges in mind, we’ve built out a new feature to supplement the quality assurance process at food facilities. Placement QA will allow food companies to see images of every meal assembled by Chef robots (and down the line by human depositors too) in their facilities, highlighting the placement of each ingredient deposit within each meal’s container. Utilizing computer vision, Chef systems track containers on a production line and capture un-occluded images before and after food has been plated within them. These images are stored in Chef’s database, and a library is created for each food facility customer that they can access at any given time.
The feature provides food companies with an unprecedented ability to retain imagery data on every single meal assembled at their facilities. We already provide food companies with data on “pick weights” (the weight of each ingredient deposit); now, food companies can combine pick weight data with real-time imagery to paint a full picture of the quality of each meal assembled. Further, the feature includes orientation detection so no matter the position of a container on an assembly line, our software will automatically reorient the image to create a uniform view across all images in a library.
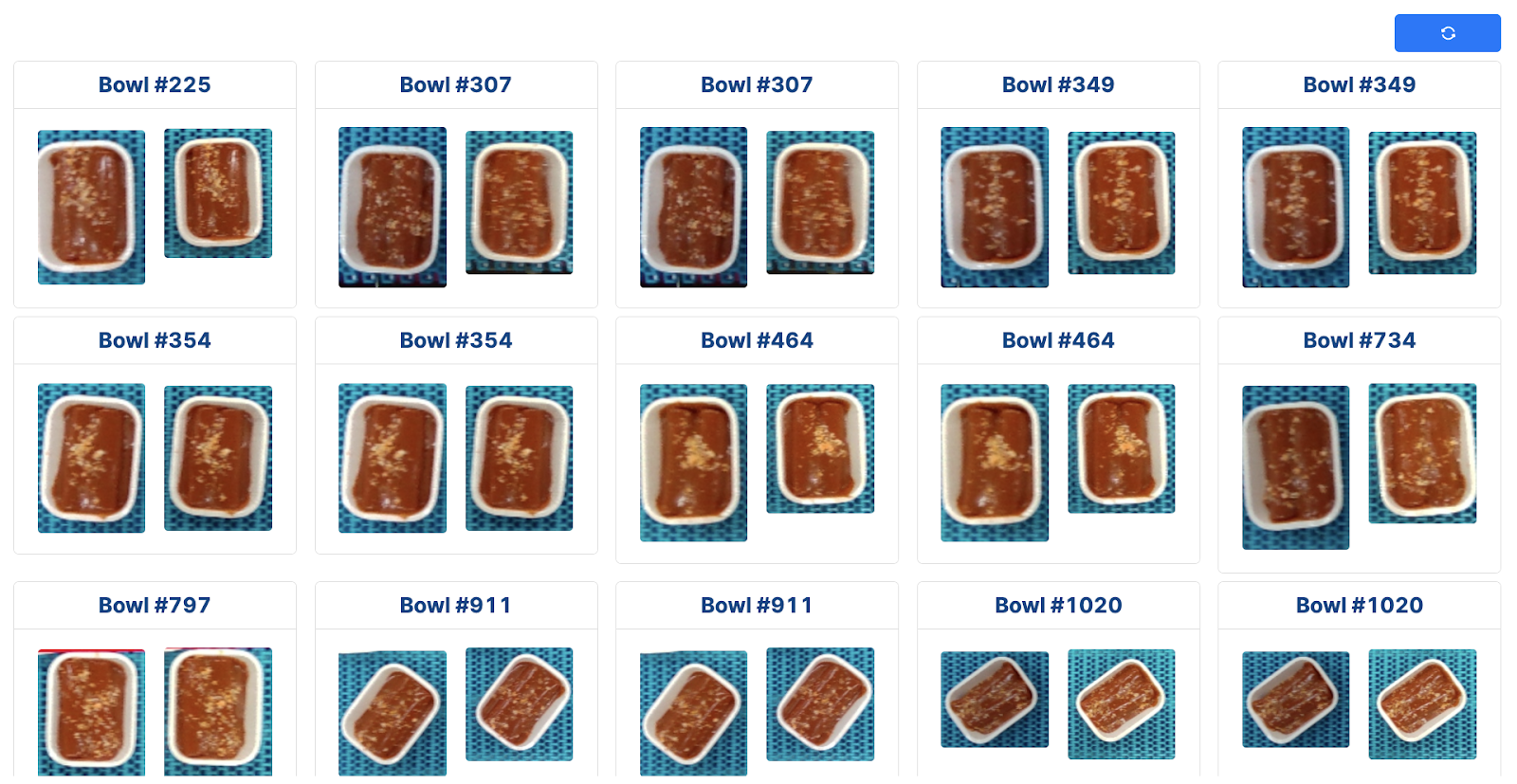
Placement QA provides big advantages to quality control teams. In the event of an incident like metal or plastic contamination, companies can browse their image library by timestamp and pinpoint the exact time an incident has occurred. This allows them to isolate the occurrence of an incident to a specific timeframe and inspect meals that may have been affected accordingly, saving them from the expense associated with having to throw out an entire production run’s worth of meals and further reducing labor overhead as a direct result of reducing the number of employees needed to conduct spot checks.
Typically, food facilities select meals on a line at random to inspect. Placement QA enables food companies to now complete computational QA for every meal produced. This gives them the ability to assess each meal across pick weight and image data metrics, setting scores for each ingredient deposit. Scores can be based on factors like whether the food was placed, how accurately it was placed within the proper compartment of a tray, and the quality of how food was spread or clumped.
Placement QA generates measurable ROI for food companies. Along with enabling companies to take a more targeted approach to quality control and thus sparing them the expense of having to dispose of large batches of meals, Placement QA also provides the benefit of being able to more closely monitor variables like product consistency and food giveaway. The ability to inspect data on these variables across every meal assembled provides companies with the insights needed to adjust processes to maximize output and minimize waste, ultimately increasing yields for the company.
Beyond the quality control and ROI benefits Placement QA creates for customers, the feature also serves to improve our AI models at Chef. The more data we accumulate, the more our AI models learn through the process of continuous improvement. With over 25 million servings produced already and this figure increasing exponentially, we own a massive data set of meal imagery, allowing our customers the best performance possible.